Enhancing Recommender Systems with Cross-Attribute Matrix Factorization and Shared User Embeddings
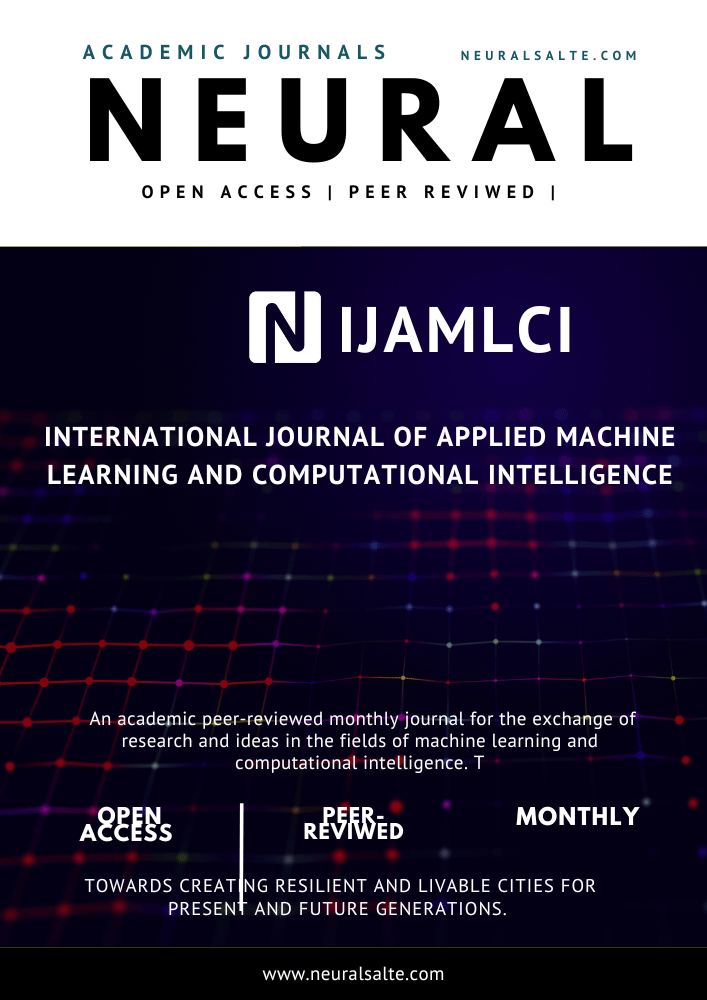
Abstract
This paper introduces an advanced model for recommender systems, combining cross-attribute matrix factorization with shared user embeddings. This hybrid approach is designed to improve recommendation quality, particularly in scenarios with sparse data and for new users or items. By incorporating both user and item attributes into the matrix factorization process, our model effectively addresses the cold-start problem and increases the robustness of recommendations. Extensive evaluations on the MovieLens and Pinterest datasets confirm the superiority of our model, highlighting its potential for practical applications in diverse recommender system environments.