Evaluating the Impact of Uncertainty in Big Data Analytics: Case Studies and Challenges
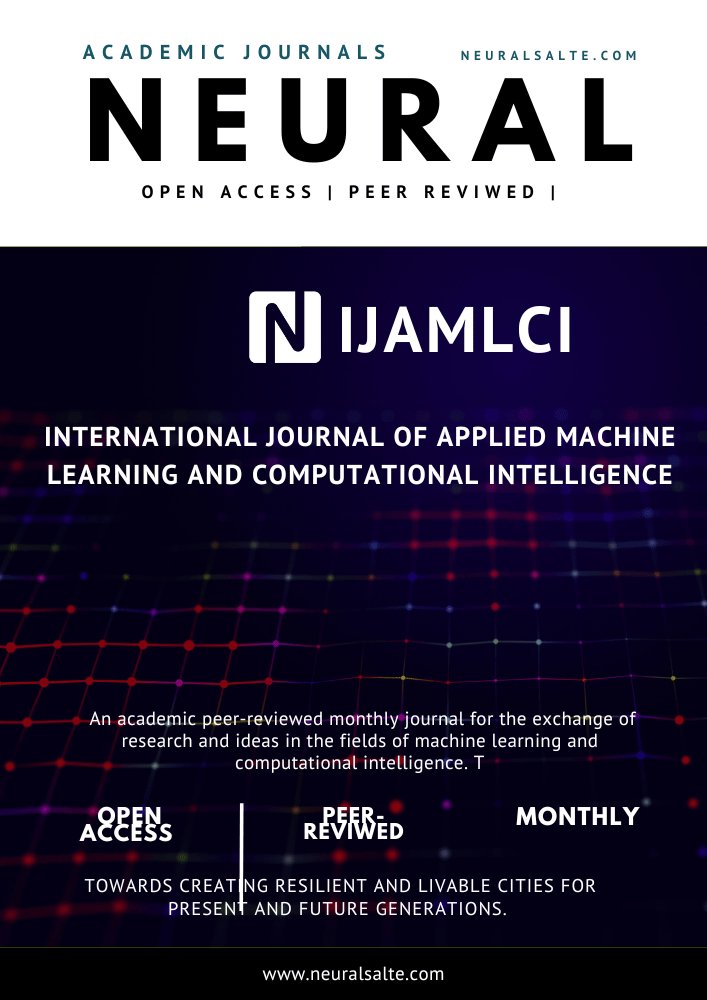
Abstract
The booming field of big data analytics has attracted significant attention in both the academic and business worlds, driven by the need to extract useful insights from ever-growing and complicated data sets. The volume of data collected in numerous areas such as healthcare, social media, urban infrastructure, agriculture, finance, and education has increased due to developments in sensor networks, cyber-physical systems, and Internet of Things (IoT) technologies. However, these data are often questionable due to noise, incompleteness, and inconsistency, making their interpretation difficult. To systematically examine large amounts of data and enable highly accurate data-driven decision making, sophisticated analytical approaches are required. As data characteristics such as volume, variety and velocity increase, the level of inherent uncertainty also increases, reducing confidence in the subsequent analysis and decisions. Artificial intelligence (AI) approaches, which include machine learning, natural language processing, and computational intelligence, have demonstrated improved performance in terms of accuracy, speed, and scalability in big data analysis compared to traditional methods. Existing research largely focuses on specific approaches or areas; Nevertheless, there is a significant lack of studies addressing the difficulty of uncertainty in big data and the role of AI in minimizing this problem. The purpose of this article is to provide a complete review of the literature on big data analysis and outline the outstanding difficulties and future prospects for managing and mitigating uncertainty.
Keywords
Big Data, Uncertainty, Artificial intelligence, computational intelligence, Natural Language Processing
Author Biography
Ahmed Khan
Rubab Naveed