Optimizing Audience Segmentation Methods in Content Marketing to Improve Personalization and Relevance Through Data-Driven Strategies
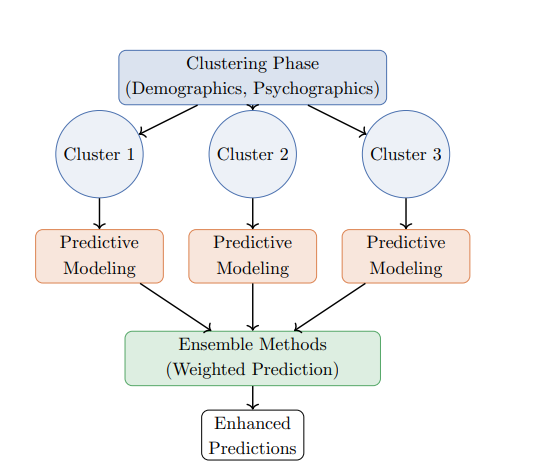
Abstract
Personalization and relevance in content marketing relies heavily on audience segmentation. This paper investigates and optimizes audience segmentation methodologies using data-driven strategies, presenting a detailed examination of various segmentation techniques, including demographic, psychographic, behavioral, and predictive segmentation. With advancements in data analytics and machine learning, segmentation is increasingly reliant on large-scale data, enabling precise consumer profiles. The study also examines the role of clustering algorithms, such as \(k\)-means and hierarchical clustering, and probabilistic models to categorize consumer segments effectively. Through mathematical modeling, we evaluate the accuracy of these methods, proposing optimizations to existing segmentation approaches by incorporating hybrid models. Our results indicate that enhanced segmentation accuracy can be achieved through a combination of supervised and unsupervised learning algorithms, offering improvements in targeted content delivery. The implications of these findings are significant, in terms of reducing audience alienation and increasing engagement rates. This study offers a quantitative analysis of segmentation accuracy metrics, such as adjusted mutual information (AMI) and silhouette score, to evaluate segmentation model efficacy. The results suggest that incorporating hybrid segmentation methods that leverage both behavioral and predictive models yields a marked improvement in personalization. Consequently, this approach not only enhances consumer satisfaction but also optimizes resource allocation in content marketing campaigns.