Fraud Detection in eCommerce Payment Systems: The Role of Predictive AI in Real-Time Transaction Security and Risk Management
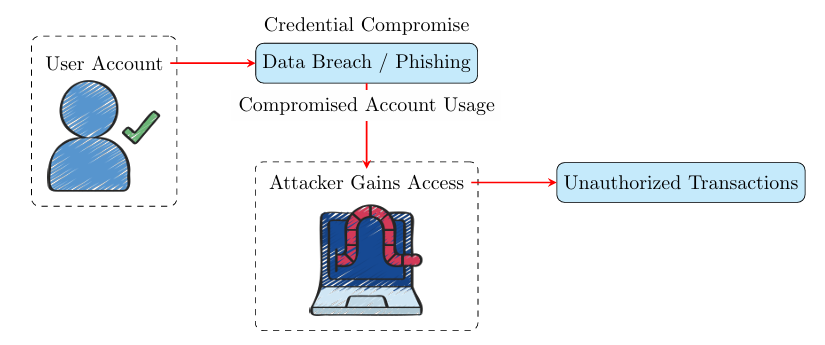
Abstract
Ecommerce platforms need to assure transaction security in the face of rising challenges and fraud attempts that are perilous for business and consumers alike. Predictive AI has become a quintessential tool for fraud detection in real time in these systems. Traditional rule-based fraud detection methods have tended to be brittle, allowing little room for adaptation as the nature of fraud changes; ML models scale dynamically for detection of threats. These models analyze huge datasets, find anomalies, and flag possible fraud activities, thus enabling systems to make autonomous decisions during the process of payment. Real-time analysis by AI reduces latency in fraud detection; hence, security is increased with minimal disturbance to real transactions. Besides choosing suitable models, predictive AI implementation involves feature engineering to optimize data and deployment in production environments. This paper addresses the integration of both supervised and unsupervised learning techniques for fraud detection in eCommerce payment systems, with a contributing role of AI in relation to data privacy, improvement of customer authentication, and continuous learning with respect to emerging cyber threats. Thus, this research has sought to explore how eCommerce payments in cybersecurity are being remade by predictive AI, which is comprehended through the operational mechanisms and possible implication of such AI models.
Keywords
AI models, eCommerce, fraud detection, predictive AI, real-time analysis, supervised and unsupervised learning