Data-Driven Decision-Making in Healthcare Through Advanced Data Mining Techniques: A Survey on Applications and Limitations
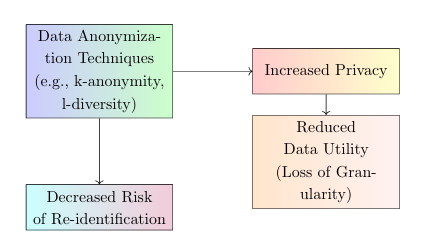
Abstract
Data-driven decision-making (DDDM) is playing a growing role in healthcare, aiming to enhance patient outcomes, increase efficiency, and reduce costs. The increase in medical data—such as electronic health records, medical imaging, and genetic information—provides opportunities for more accurate diagnoses and personalized treatments. With the exponential growth in medical data and more—advanced data mining techniques have become useful tools for extracting actionable observations. This influx of complex and varied data offers opportunities for more precise decision-making, but it also presents significant analytical challenges. Advanced data mining techniques have been developed to handle these complexities, enabling healthcare providers to extract meaningful patterns and observations from large datasets. These tools allow for the extraction of useful patterns that might otherwise remain hidden, guiding clinical and operational decisions. These methods support clinical decision-making, patient management, and operational optimization, enhancing outcomes while addressing efficiency. Successful implementation requires addressing data integration, privacy regulations, and model interpretability issues. This paper discusses the applications of data mining in healthcare decision-making, discussing how these methods are applied to predictive analytics, personalized medicine, resource management, and early disease detection, while also identifying the challenges involved in their adoption.
Keywords
data integration, data mining, healthcare analytics, personalized medicine, predictive analytics, wearable devices