Developing Interpretable and Explainable AI Models for High-stakes Decision Making in Societal Contexts
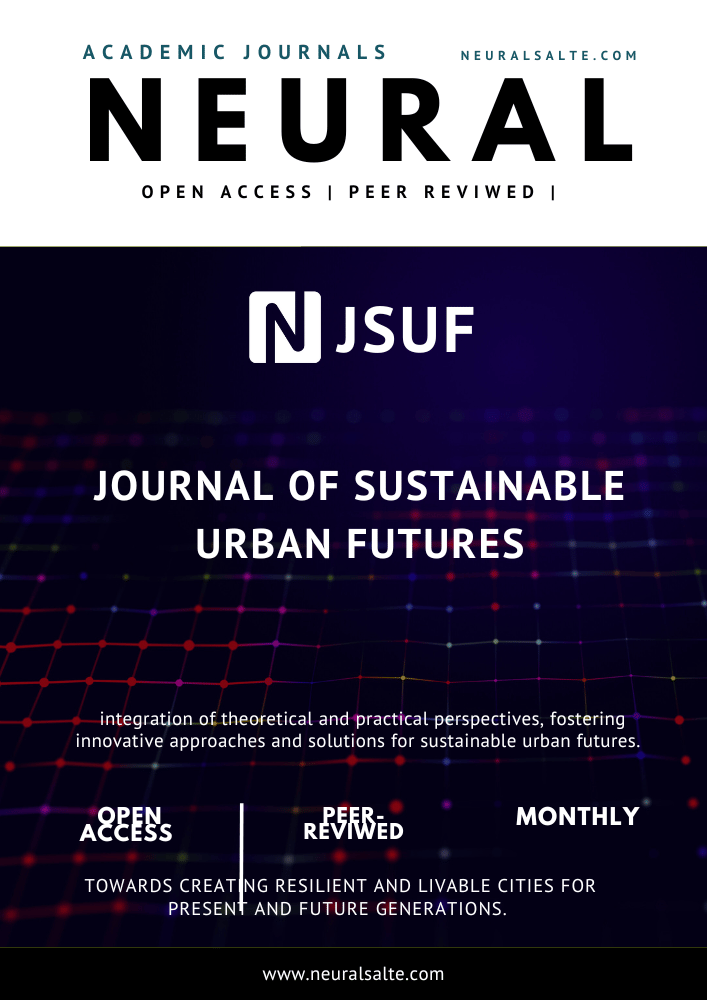
Abstract
As artificial intelligence (AI) systems become increasingly integrated into high-stakes decision-making processes in societal contexts, the need for interpretable and explainable AI models has become paramount. This research paper explores the challenges and opportunities associated with developing AI models that are transparent, understandable, and accountable, particularly in domains such as healthcare, criminal justice, and financial services. We discuss the limitations of current black-box AI models and highlight the importance of interpretability and explainability in building trust and ensuring fairness in AI-assisted decision-making. We review state-of-the-art techniques for developing interpretable and explainable AI models, including rule-based systems, decision trees, and attention mechanisms, and compare their strengths and weaknesses in different societal contexts. We also propose a framework for evaluating the interpretability and explainability of AI models, taking into account factors such as model complexity, domain expertise, and stakeholder requirements. Finally, we discuss future research directions and emphasize the need for interdisciplinary collaboration between AI researchers, domain experts, and policymakers to ensure the responsible development and deployment of interpretable and explainable AI models in high-stakes societal contexts.
Author Biography
Hoang Minh Chau
Hoang Minh Chau, Department of Law, Lao Cai College, 8 Le Dai Hanh Street, Lao Cai City, Lao
Cai Province, Vietnam