Big Data-Driven Predictive Modeling for Pricing, Claims Processing and Fraud Reduction in the Insurance Industry Globally
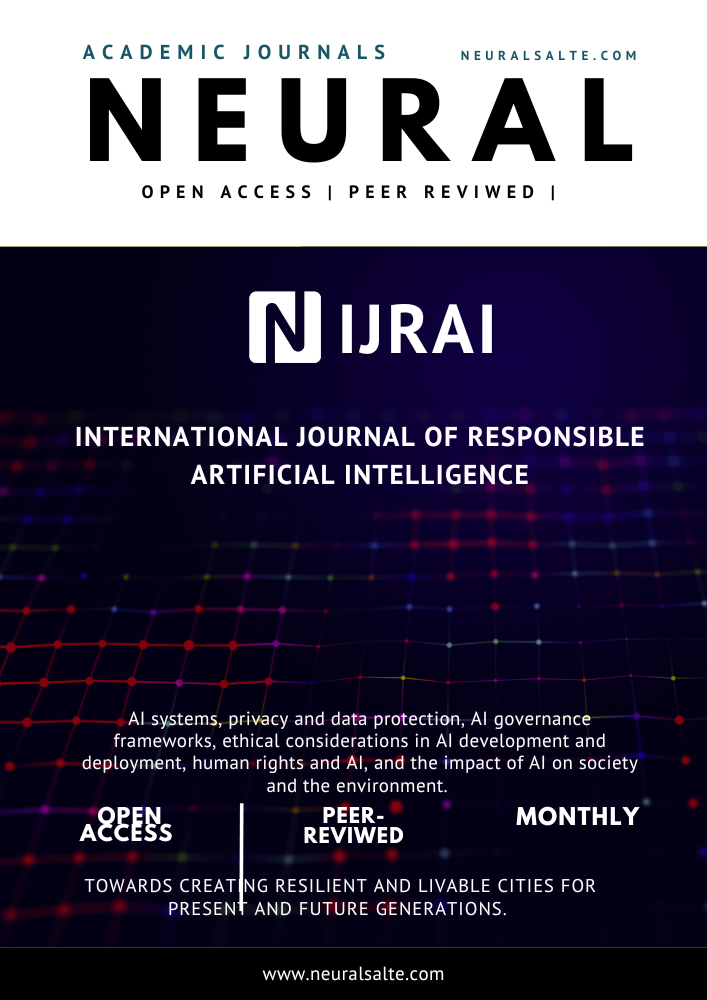
Abstract
The insurance industry has experienced massive growth in data generation and collection in recent years. Advances in technology have enabled insurers to capture vast amounts of structured and unstructured data from multiple internal and external sources. This "big data" presents tremendous opportunities for insurers to gain actionable insights, make data-driven decisions, and design innovative products and services. This paper examines the application of big data and predictive modeling techniques in three key areas of the insurance sector - pricing, claims processing, and fraud reduction. An extensive literature review explores current research and real-world examples of how leading global insurers are leveraging big data analytics to price policies more accurately, speed up claims processing, detect fraudulent claims, and reduce losses. The adoption of predictive modeling techniques based on machine learning algorithms to unlock insights from big data is a predominant theme. The paper also discusses some of the main challenges faced by insurers in implementing big data initiatives, such as data quality, lack of analytical talent, legacy IT systems, data privacy, and organizational resistance. Recommendations are provided for insurers looking to harness big data analytics to enhance competitiveness, improve risk assessment, deliver superior customer experiences, and strengthen fraud detection.
Keywords
Big data, Predictive modeling, Machine learning, Pricing, Claims processing
Author Biography
Nguyen Van Anh
Tran Minh Duc