Deep Learning Assisted Strategies for Minimizing Radiation Exposure During Computed Tomography Angiography Procedures
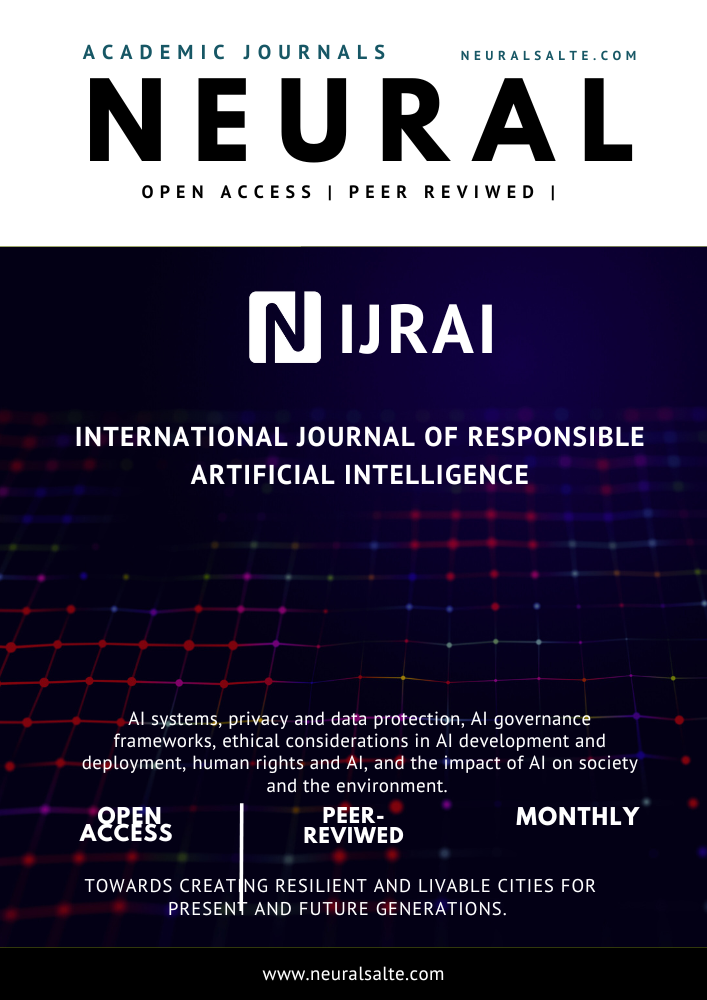
Abstract
Computed tomography angiography (CTA) procedures are crucial in medical diagnostics, yet the radiation exposure poses serious safety risks to both patients and healthcare practitioners. The utilization of deep learning, a prominent subfield of machine learning, offers innovative solutions for these challenges. In this paper, we explore deep learning's role in optimized image reconstruction, including iterative reconstruction techniques and denoising autoencoders, to create high-quality images with reduced noise and radiation. Adaptive dose modulation, assisted by deep learning models, can predict the required radiation dose based on individual patient characteristics, minimizing exposure. Image augmentation techniques enable the synthesis of missing data from reduced-dose scans, maintaining image quality without additional radiation. Deep learning's predictive models aid in the precise real-time positioning of patients, and facilitate radiation dose tracking and feedback, personalized protocols, and real-time dose estimation. The use of virtual monochromatic imaging in dual-energy CT is enhanced through deep learning, enabling optimized reconstructions with potential radiation dose reduction. The paper also highlights the potential of transfer learning and data augmentation in addressing the challenges of training deep learning models with limited datasets. Deep learning-enabled simulations and phantom studies assist in the pre-implementation validation of dose-reduction strategies. Personalized scanning protocols, driven by deep learning, provide the possibility of patient-specific optimization for image quality and radiation reduction. The integration of these strategies signifies a substantial advancement towards safer and more efficient CTA procedures. However, success demands continuous validation, specialized training, and cohesive collaboration between radiologists, physicists, and AI specialists to guarantee the optimal performance, safety, and efficacy of these cutting-edge methods.
Keywords
Optimized Image Acquisition, Image Augmentation and Synthesis, Real-time Feedback and Personalization, Cumulative Dose Management, Training and Validation Enhancements
Author Biography
Gayatri Tiwaskar
Gayatri Tiwaskar
Customer Pharmaceutical Quality Assurance, Dadasaheb Balpande College of Pharmacy,Besa,Nagpur, Maharashtra, India.
ORCID: 0000-0001-9672-4385
Sara Yasmin El-Mahmoud
Sara Yasmin El-Mahmoud
Computer science department, Damanhour University, El Gomhoria Street, Damanhour, Al Beheira Governorate, Egypt